In this blog, we explore the concept of AI-Ready Data, what it means, why it’s important and what steps you can take to make your data AI-Ready.
AI-Ready Data is required when you want to ‘tune in’ AI to your organisation’s specific needs. Knowledge Management disciplines are becoming increasingly important within organisations wanting to achieve tailored results from their AI investment.
Why is AI-Ready Data Important?
Your data is how your business differentiates. Using an out-of-the-box AI capability will keep you alongside your competitors; however, you are unlikely to stand out in your market.
To fully benefit from what AI can offer, your data is the secret sauce.
Knowing how to make your organisation’s data available for AI and tuning in to your needs is becoming a critical success factor.
In the 2023 Gartner IT Symposium Research Super Focus Group, only 4% of respondents said their data is AI-ready. While 37% said they are well-positioned to have AI-ready data, 55% reported that “it will be difficult”.
In addition, a recent survey of 334 Chief Data Officers (or equivalent roles) highlighted Data Quality as the biggest challenge to realising value from Generative AI, alongside finding the right use cases. 93% agreed that data strategy is crucial for getting value out of Generative AI. [Survey sponsored by Amazon Web Services (AWS) and conducted in collaboration with the International MIT Chief Data Officer and Information Quality (MIT CDOIQ) Symposium. Also referenced in Harvard Business Review.]
Shifting Data Management Requirements
AI-Ready Data isn’t a new concept. Efforts to create good quality data have supported AI in areas such as predictive analytics over a number of years. What is changing is the type of data involved as we move into Generative AI being far more accessible and pervasive (ChatGPT, Gemini, Anthropic, Grok etc.).
In this context, we can broadly split data into two types: structured and unstructured.
Structured data follows a well-defined layout, as you would find in a database that supports operational systems and includes, for example, sales transactions.
Unstructured data doesn’t conform to a standard layout and includes text, documents, and images. The variety within unstructured data leads to complexity in handling it, but it is often where the richness lies that AI can exploit.
Organisations will typically focus more on managing and maintaining their structured data, as the benefits of doing so are more directly linked to creating business value. For example, maintaining a single and enriched view of customers, tracking all sales transactions, and knowing how many staff are employed.
Unstructured data is generally unloved. Think about maintaining documents and versioning in SharePoint sites. How well-maintained and accessible are policy documents on your intranet sites? What about all those PDF-scanned contracts or even hard-copy documents sitting in filing cabinets?
Maintaining this type of data has been labour-intensive, and it is often difficult to draw a straight line to business value, hence its unloved status in many organisations.
There is now a much greater need to manage both your structured and unstructured data to tune in AI for your organisation:
- Emails to train AI on sentiment analysis
- Previous commercial contracts for law firms to be able to query
- Consistent branding for marketing
- Addressing potential bias in an underlying model
We are going to see a much greater focus on knowledge management to support AI initiatives.
Tuning-In AI
As previously mentioned, AI has been used for many years. Structured data has driven predictive analytics to support areas such as sales and supply chain forecasting. In this area, huge effort has been put into ensuring the quality of data to train models to make predictions accurately. This sometimes includes real-time data for dynamic pricing and in-day supply chain decisions.
Computer Vision is an example of unstructured data being used for predictive analysis – again huge effort has been put into sourcing and labelling images for training a model. For example, identifying products on a shopping shelf, Amazon Go or medical image reporting.
With Generative AI, where there are benefits for every business, the impact is far more pervasive. The risk of being left behind is much greater. This leads to a general need for all businesses to consider how to tune in AI to benefit their organisation.
But how do you tune in Generative AI for your business – the table below provides the key approaches and the data management considerations:
Using the analogy of learning a language we introduced in our blog about Business-Ready Data:
- Prompt Engineering is like learning set phrases to use in specific situations.
- RAG is having access to a dynamic, constantly updated dictionary or encyclopaedia that you can consult at any time.
- Fine-tuning is similar to taking advanced lessons to refine your language skills for subjects where particular words and phases are required.
- RLHF mirrors the process of practising your language skills in real conversations and refining your understanding based on feedback.
- Training from scratch is learning a new language in a way that’s completely tailored to your needs and pace.
As with language proficiency, managing your data to be successful with AI is not a one-off activity but a continuous cycle of learning and improvement.
The choice of approach will need to be carefully considered and determined by your specific needs. It highlights the importance of data and knowledge management to ensure data is well-categorised, searchable and regularly updated. This is particularly important for RAG and fine-tuning, where the accuracy and relevance of data directly impact AI performance.
Defining AI-Ready Data
We use the following Data Principles to help describe what shape your data needs to be in to maximise the value you get from investment in digital, data and AI solutions.
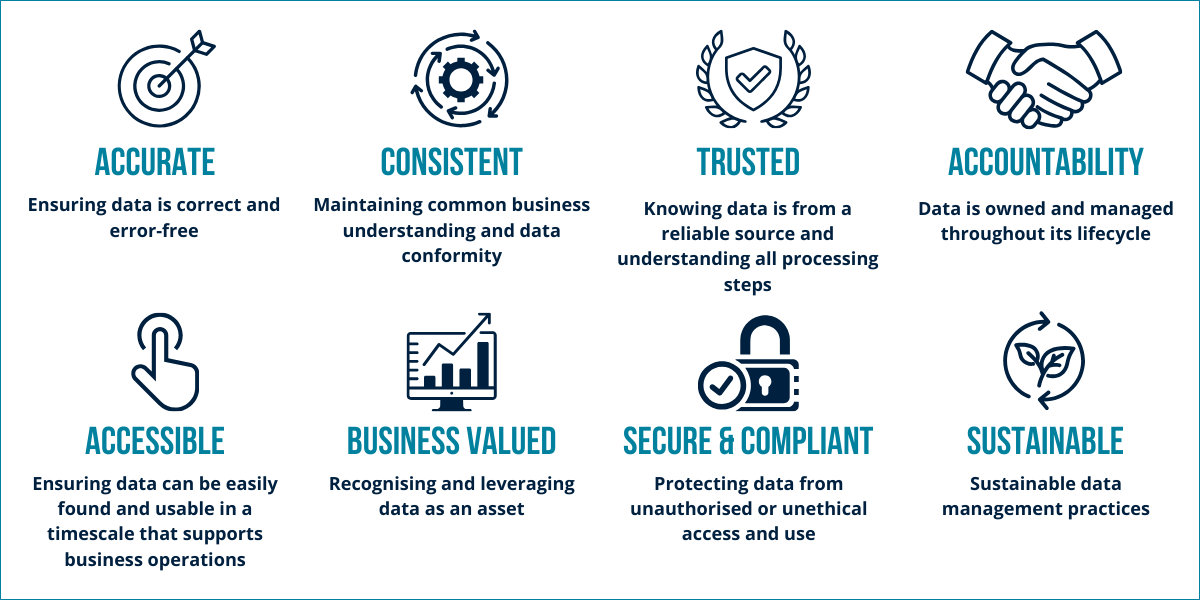
ReachDDG Data Principles
Based on the previous description of how your organisation’s data can enhance your AI capability, we can apply these principles to describe AI-Ready Data. This approach focuses on starting with a base model rather than starting from scratch.
Data Principle: Accurate
-
- Accurate data is needed for reliable and useful outputs from AI models.
- Accuracy covers typical data quality measures for structured data. Plus, when looking at accuracy within unstructured documents, you will also need to consider the volume and diversity of inputs, in particular with fine-tuning.
- Consider how you will keep your data up to date and regularly test for accuracy.
Data Principle: Consistent
-
- Consistency is important to ensure AI appropriately interprets your intentions and reduces the scope for ambiguity.
- In terms of unstructured data, this will include consistent terminology, style, and branding. For example, if you’re looking at training a Chatbot in a preferred style, there would need to be consistency in style across all inputs. Training an AI in legal terminology would require consistency in language across the documents made available.
Data Principle: Trusted
-
- Data should come from known and reputable sources, both internal and external. Clear lineage will be required, which can be supported by knowledge graphs.
- There will be potential ethical and legal considerations in the data being used, so stay aware of the evolving AI legislative landscape.
- You may also want to consider how the underlying model has been created in order to address any concerns upfront.
Data Principle: Accountability
-
- Each organisation requires clear ownership and accountability for the data feeding into the AI – ownership of content and accountability for maintaining that data, whatever its structure, across the whole data lifecycle from creation to deletion.
- Clear policies and governance will be needed.
Data Principle: Accessible
-
- Data must be structured and stored in a way that’s easily accessible to AI systems; consider cloud-based solutions for scalability and flexibility.
Data Principle: Business-Valued
-
- AI use cases should be aligned with business goals
Data Principle: Secure & Compliant
-
- Strong security and compliance measures are essential to protect sensitive data used by AI from breaches or misuse.
- Various industries will have legal requirements, and Governments are beginning to introduce legislation that you should maintain awareness of
Data Principle: Sustainable
-
- This is a challenging principle in the context of AI, which inherently has a significant (and largely unquantifiable) impact on sustainability.
- Continue to be considerate of the impact, and explore how you can minimise it.
There are two key points to call out:
1. Data volume – one of the key challenges will be creating and maintaining sufficient good quality data. Whilst at a different scale, a recent WSJ Article (“For Data-Guzzling AI Companies, the Internet Is Too Small”) highlights the importance of good quality data to feed AI models.
This isn’t just about data volumes; poor-quality data will have a detrimental impact on AI performance.
2. Knowledge Management – by far, the most crucial factor will be how organisations adapt their Data Management approaches to address the additional challenges posed by tuning in AI for your organisation, particularly unstructured data. It should be possible to create a virtuous circle where AI can be used to improve knowledge management, which in turn enhances AI effectiveness for your organisation, for example, automated meta-data generation and helping create knowledge graphs.
Be Focused and Start Small
It’s important to start with focused use cases that add clear value to your organisation. Understand what AI-Ready Data means for your organisation and focus on developing your Knowledge Management capability.
Develop a clear view of how you will manage and maintain your data to help AI give your organisation an edge.
If you need help with any of the items discussed in this blog, please contact us.